Data Mining Techniques in Healthcare Decision System
In the last decade, various methods have been used to explore and find patterns and relationships in healthcare data. But from the last few years, data mining was exploring more in the sector of health. As data mining showed some promise in the use of its predictive techniques to improve the delivery of human services. As the Big Data movement has gained momentum over the past few years, Organization taking interest in the use of various techniques and methods to analyze the big data related to health issue. We study different techniques & applications for the evaluation of hospitals, relationship with patients & their treatment. This article gives us a brief about how data mining can change our overall healthcare department & improve the health of the peoples.
- INTRODUCTION: Data mining is useful for extracting information. Data mining is used for commercial and research purposes. In this paper we mainly discusses the operations of information extraction in the various field of health. In this work, a brief survey is carried out on the applications & uses of data mining in the health issue. Data analysts algorithms are applied in medical industry which is useful for finding old, new & common disease occur in the common peoples. Various applications are found in this area such as pharmaceutical & hospital management. The extracting information is helpful for developing an understanding of the operation domain, selection & creation of data, processing & transformation. Data Mining has been used in a variety of function such as marketing, customer
relationship management, engineering, and medicine analysis.
- DATA MINING: Information from large data, as it is also known is the non-trivial extraction of implicit, previously unknown and potentially useful
information from the data. This encompasses a number of technical approaches, such as clustering, data summarization, classification, finding dependency networks, analyzing changes, and detecting anomalies.
- DATA MINING PROCESSES: There are various stages in extracting the information i.e.
3.1 Selection: The first stage is selection of data in which data is collected from different sources
3.2 Preprocessing: The second stage is preprocessing the data that is collected.
3.3 Transformation: The third stage is transformation of data into suitable format for their further processing
3.4 Data mining: The fourth stage includes data mining where suitable Data Mining technique is applied on the transformed data in order to extract valuable information.
3.5 Interpretation & evaluation: The data generated from fourth stage are evaluated. The evaluation helps to discover knowledge from large data that will be useful for decision making.
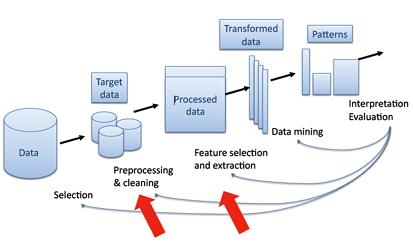
Fig 1: Data Mining Process
- DATA MINING TECHNIQUES IN HEALTH CARE: In data mining there are mainly two types of learning techniques. The two methods are supervised learning & unsupervised learning.
- Supervised Learning Techniques: Supervised learning involves a person that helps to learn. The learning predicts an outcome based on certain criteria. Examples of such learning are classification.
- Unsupervised Learning Techniques: Unsupervised learning is a technique that does not involves a person. It’s a branch of machine learning that learn from test data. e.g. clustering.
- Data Mining Models: Models are mainly classified into two broad categories:
- Predictive model
- Descriptive model
5.1 Predictive model: Predictive modeling is a process that uses data mining and probability to forecast outcomes. Predictive models are used for the results. When results are simulated then statically model are built.
5.2 Descriptive model: The descriptive model recognizes the designs or relationships in data and discovers the properties of the data studied. For instance, Clustering, Summarization, Association rule, Sequence discovery etc
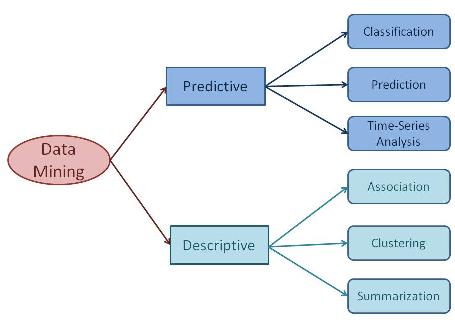
Fig 2: Data mining Models
- Data mining Task
6.1 Summarization: In summarization, the arrangement of information is preoccupied that outcomes into a littler arrangement of information which gives us a general audit of the information.
6.2 Association: Association also has great impact in the health care industry to discover the relationships between diseases, state of human health and the symptoms of disease. An integrated approach of us association and classification also improved the capabilities of data mining. By using this rule effective results are generated.
6.3 Classification: Classification comprises of two footsteps: - 1) Training and 2) Testing. The accuracy of classification model hinge on the degree to which classifying rules are true which is estimated by test data.
6.4 Clustering: Clustering is different from classification; it does not have predefined classes. Clustering algorithms discovers collections of the data such that objects in the same cluster are more identical to each other than other groups.
6.5 Trend analysis: We can watch a great deal of time subordinate information in writing. Such information can be seen as items with a 'period' trait.
6.6 Regression: Relapse is taking in a capacity which can outline information thing to a genuine – esteemed expectation variable. Its a utilized procedure for expectation.
- DATA MINING APPLICATIONS IN HEALTHCARE SECTOR: Medical industry today generates large amounts of complex data of patients, hospital resources, disease diagnosis, electronic patient records, medical devices etc. Larger amounts of information are a key resource to extract the data for cost-savings and decision making. Data mining operations in healthcare can be grouped as the evaluation into broad categories.
- Diagnosis and prediction of diseases – When it comes to social insurance businesses, conclusion and anticipation of ailments is imperative, it is a standout amongst the most imperative motivation behind utilizing information digging for social insurance.
- Effective treatments – By contrasting components like causes, indications, symptoms and cost information mining is utilized to break down the adequacy of medicines. For instance, one can look at the consequences of medications of various patients which were experiencing a same diseases yet were treated with various medications. Along these lines, we can discover which treatment is compelling regarding the patient's wellbeing and cost.
- Healthcare management: To aid healthcare management, data mining applications can be developed to better identify track chronic disease states, high-risk patients, design appropriate interventions.
- Customer relationship management. While customer relationship management is a approach in managing interactions between commercial organizations—typically banks and retailers—and their customers, it is no less important in a healthcare context.
- Reduction in insurance fraud and abuse–Human services guarantor develops a model to distinguish abnormal examples of cases by patients, doctors, clinics, and so on.
- Medical Device Industry: Medical devices are very important to healthcare department. If devices help us to make better decision in our life and also help us to improve the health of the people.
- Limitations of Data Mining: Although data mining is a very powerful tool, but to be successful, data mining needs a skilled user who will supply the correct data. If the user supplies incorrect or minimal amount of information, output will be affected & forecast will not be credible.
- CONCLUSION: In this paper, we have talked about that data mining techniques, applications used in medical organization. There is a rapidly change in the volume of restorative information, data mining methods have high utility in this field. Different assignments are broke down inside the domain of human services associations. This paper investigates distinctive strategies, their points of interest and disadvantages. Maybe, there is no single information mining strategy which can give reliable information from a wide range of social insurance information. In fact, the execution of strategies shifts from one dataset to other dataset. For continue use of these procedures in medicinal services space, there is a need to upgrade and secure wellbeing information sharing among different gatherings. Further, as medical data are not limited to just quantitative data, such as physicians’ records, it is necessary to also explore the use of text mining to expand the scope and nature of healthcare. In this paper we make a contribution to the data mining and healthcare literature and practice. It is hoped that this paper can help all parties involved in healthcare & the benefits of healthcare data mining.